Cybersecurity analysts often rely on Google to find relevant information while performing analysis. For many industry verticals Large Language Models (“LLMs”) have replaced the need for Google by directly providing the answer versus the previous status quo of “you figure it out with these 10 blue links”. However, for cybersecurity workloads, LLMs typically fall short of expectations due to how quickly the cybersecurity landscape changes. An LLM’s training set is often several months old at a minimum, meaning it lacks recent cybersecurity information and insights, frequently leading to hallucinations and inaccurate responses.
How can we enable cybersecurity analysts to confidently use and trust LLMs with cybersecurity inquiries?
In this blog, we’ll explore the current shortcomings of using LLMs for cybersecurity use cases and why we believe building expansive Retrieval Augmentation Generation data pipelines is crucial for improving agentic cybersecurity workloads.
Using LLMs to Analyze CVE-2024-4577
To demonstrate an example of how LLMs fall short, we’ll explore a common vulnerability analyst workflow by analyzing CVE-2024-4577, a PHP Remote Code Execution vulnerability added to the National Vulnerability Database on June 12, 2024 with a CVSS 3.0 base score of 9.8. In July 2024, numerous vendors and independent researchers published blogs and articles describing in-the-wild exploitation activity affiliated with this vulnerability.
Let’s test how a popular LLM describes CVE-2024-4577. For this first example we’ll use OpenAI’s ChatGPT o1 LLM, a model publicly released in December 2024 specifically built for complex reasoning tasks and questions.

OpenAI’s o1 replies that it has no knowledge of CVE-2024-4577. To OpenAI’s credit, the above response is actually an improvement from previous GPT models that would hallucinate and reply with an answer referencing a completely unrelated vulnerability. At least we’re moving in the right direction!
The above screenshot was taken in March 2025, nearly ~8 months after this vulnerability was initially reported to NVD. The o1 model was released for limited use by OpenAI in September 2024 and fully released in December 2024. A timeline of important dates can be seen below.
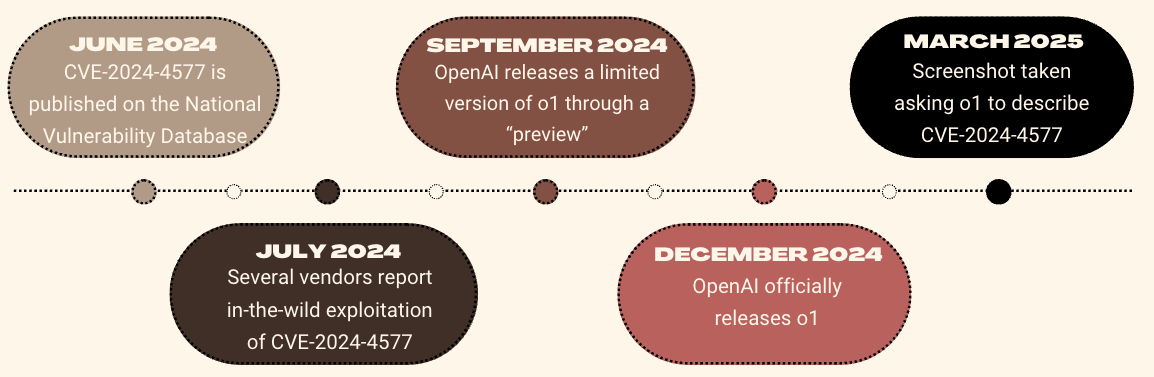
I often describe cybersecurity as a big game played between attackers and defenders. When a vulnerability is disclosed, there’s often a race between defenders to quickly remediate the vulnerability and attackers to exploit unpatched vulnerable systems. This race is often won or lost by a few hours. To consistently win, cybersecurity practitioners need LLMs to reliably reply with topical information that includes the latest updates in the cybersecurity landscape.
So why haven’t foundational model vendors solved this problem? Why can’t foundational models be trained more often?
The short answer is cost. Public reports indicate OpenAI spent 100 million to train GPT-4.0, and training costs for the recently released GPT-4.5 are substantially more, likely 500+ million. Consistently training foundation models is not financially sustainable, resulting in a majority of foundation models to be trained once every 3+ months. While this cadence will likely improve over time as compute becomes cheaper, for the foreseeable future foundational LLMs will not have up to date cybersecurity information, resulting in hallucinations and incorrect answers.
Another option to solve this problem is fine-tuning, a post-training improvement technique that is relatively cheap compared to retraining the LLM and can be completed in hours to days depending on the size of the fine-tuning dataset. This is an interesting area we’re keeping an eye on. The Google Gemini team released Sec-Gemini on April 4, 2025 that has integrations with Google Threat Intelligence (“GTI”) and other security datasets. It’s unclear from the press release if Sec-Gemini is a fine-tuned Gemini or if Sec-Gemini has built Retrieval Augmented Generation (“RAG”) pipelines to GTI on top of Gemini.
Enter Retrieval Augmented Generation
RAG was initially designed to solve the aforementioned stale LLM training dataset problem by retrieving information from a vector store and loading relevant data into the LLM context window before generating a response. To OpenAI’s, Anthropic’s, Google’s credit, and partly because Perplexity was quickly stealing their user base, many foundational model vendors have started to integrate internet search RAG into their base offering.
To demonstrate internet search RAG, we’ll use an older Open AI model GPT-4o released in May 2024 (we previously used GPT-o1) and ask “Describe CVE-2024-4577” once again. The LLM accurately recognizes that it doesn’t have the right information and indicates it’s “Searching the web” to identify online resources.
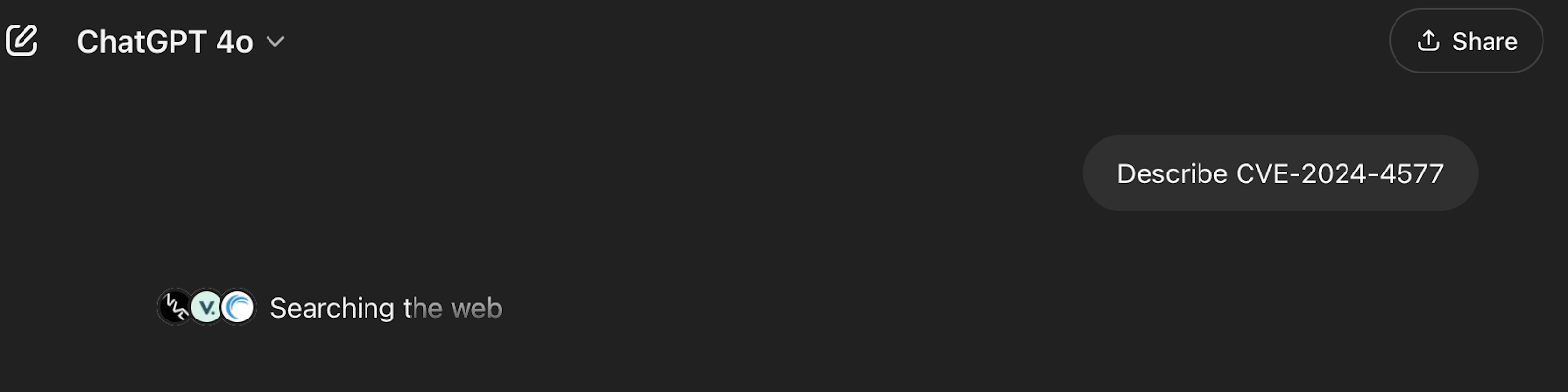
While OpenAI’s implementation is slightly a black box, we assume it’s likely feeding the retrieved articles into GPT-4o’s context window before generating the response. As the LLM response below shows, because of RAG, 4o’s output is far better than the newer o1 model designed for complex reasoning.
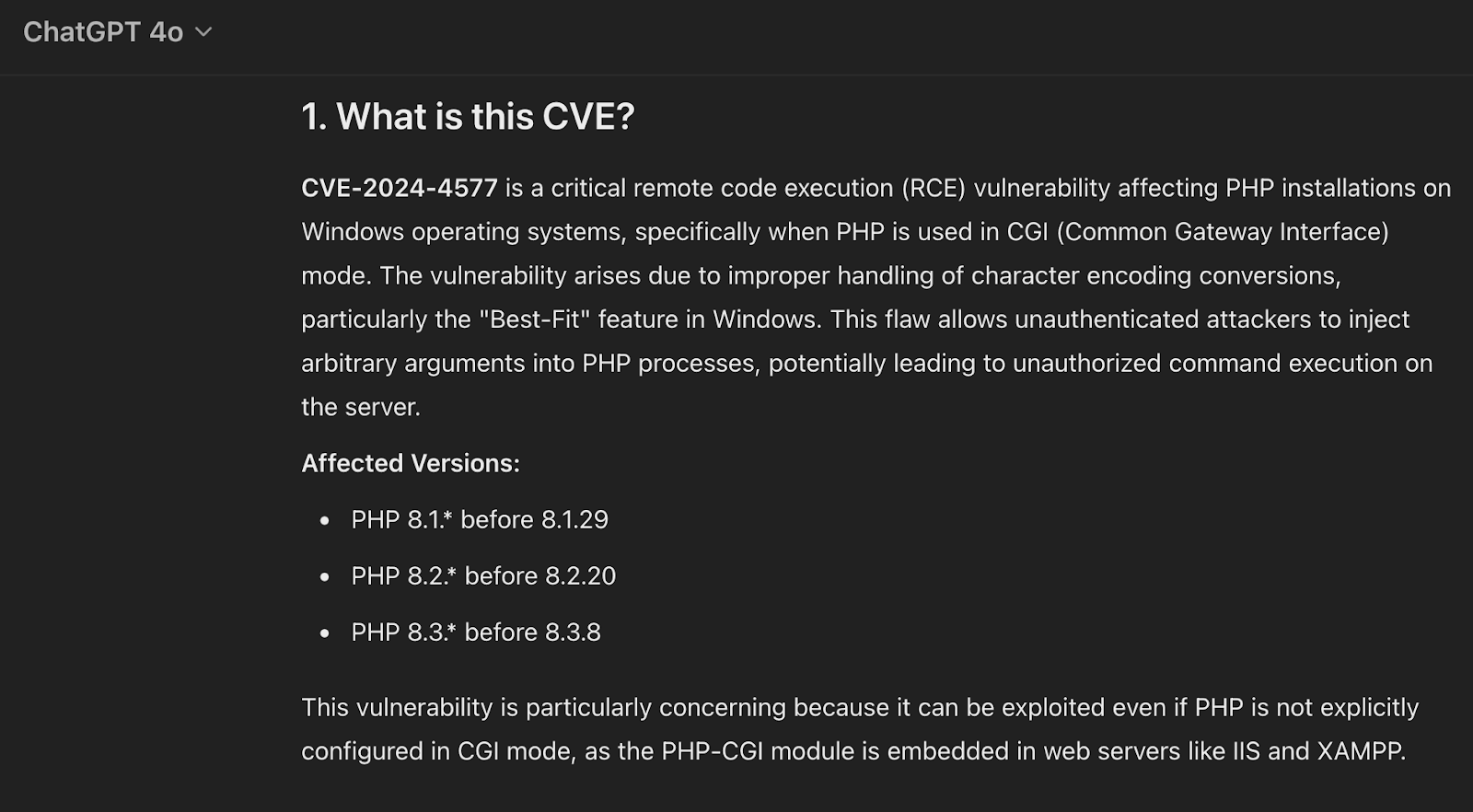
OpenAI refers to this internet search RAG technique as “web search” which essentially means a Google search is performed to identify online resources that reference the user’s question. This information is used in tandem with the LLMs training to answer the question.
Google is undeniably a great source of data, especially for third party advisories, blogs, and vendor threat intelligence reports. However, Google can miss important data including vendor advisories which are not optimized for Search Engine Optimization (“SEO”). Company incident reports can be sheepishly hidden deep within the company website, and many other vendors choose not to publicly address or disclose vulnerabilities at all as described in our previous blog.
Moving Beyond Basic Web Search
Most organizations Speculars partners with need to integrate internal data into the RAG pipeline. Cybersecurity analysts query a wide range of internal products and tools that contain important contextual data when analyzing a CVE. For example, analysts will query the CMDB entry of the affected asset, the firewall to review open ports, their proprietary threat intelligence provider for vertical specific intelligence, and SIEM to look for signs of compromise. These integrations are incredibly important to provide the LLM with updated relevant context for analysis.
How can we build these internal RAG integrations?
This space is still evolving, we believe the answer will be a combination of Model Context Protocol (“MCP”) and LLM grounding. More on how Specular is using these techniques to solve cyber security challenges soon…
Interested in discussing integrating LLMs into your cybersecurity operations? Reach out to Specular at contact@specular.ai